Predictive algorithms are no better at telling the future than a crystal ball
- Written by Uri Gal, Associate Professor in Business Information Systems, University of Sydney
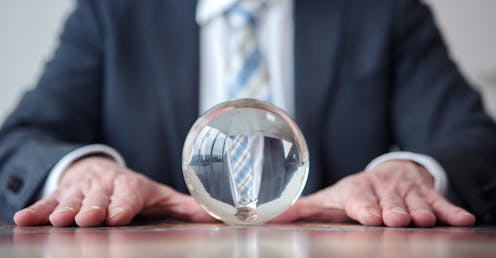
An increasing number of businesses invest in advanced technologies that can help them forecast the future of their workforce and gain a competitive advantage.
Many analysts and professional practitioners believe that, with enough data, algorithms embedded in People Analytics (PA) applications can predict all aspects of employee behavior: from productivity, to engagement, to interactions and emotional states.
Read more: Digital public: looking at what algorithms actually do
Predictive analytics powered by algorithms are designed to help managers make decisions that favourably impact the bottom line. The global market for this technology is expected to grow from US$3.9 billion in 2016 to US$14.9 billion by 2023.
Despite the promise, predictive algorithms are as mythical as the crystal ball of ancient times.
Predictive models are based on flawed reasoning
One of the fundamental flaws of predictive algorithms is their reliance on “inductive reasoning”. This is when we draw conclusions based on our knowledge of a small sample, and assume that those conclusions apply across the board.
For example, a manager might observe that all of her employees with an MBA are highly motivated. She therefore concludes that all workers with an MBA are highly motivated.
This conclusion is flawed because it assumes that past patterns will remain consistent. This assumption itself can only be true because of our experience to date, which confirms this consistency. In other words, inductive reasoning can only be inductively justified: it works because it has worked before. Therefore, there is no logical reason to assume that the next person our company hires who has an MBA degree will be highly motivated.
Read more: How marketers use algorithms to (try to) read your mind
Assumptions like these can be coded into hiring algorithms, which, in this case, would assign a weighting to all job applicants with an MBA degree. But when inductive reasoning is baked into the code of hiring applications, it can lead to unfounded decisions, adversely impact on the bottom-line, and even discriminate against certain groups of people.
For example, a tool used in some parts of the United States to assess whether a person arrested for a crime would re-offend was found to unfairly discriminate against African Americans.
They lead to self-fulfilling prophecies
Another flaw in the predictions thrown up by algorithmic analysis is their propensity to create self-fulfilling prophecies. Acting on algorithmic predictions, managers can create the conditions that ultimately realise those very predictions.
For example, a company may use an algorithm to predict the performance of its recently-hired salespeople. Such an algorithm might draw on data from standardised tests completed during their onboarding process, reviews from previous employers, and demographics. This analysis can then be used to rank new salespeople and justify the allocation of more training resources to those believed to have greater performance potential.
This is likely to produce the very results that the initial analysis predicted. The higher-ranked recruits will perform better than those ranked lower on the list because they have been given superior training opportunities.
Read more: What businesses can learn from sports about using algorithms
Calculating probabilities of future events is meaningless
Some practitioners recognise the flaws in the predictive capability of algorithmic systems, but they still see value in generating models that indicate probability.
Rather than predicting the occurrence of future events or states, probabilistic models can indicate the degree of certainty that events or situations might occur in the future.
However, here too it pays to be a little sceptical. When a model calculates that an event is likely to happen it does so as a percentage of 100% certainty. Any probabilistic prediction is only possible in relation to the possibility of complete certainty. But since complete certainty is impossible to predict, probabilistic models are of no real significance either.
Algorithms don’t ‘predict’, they ‘extrapolate’
So if they cannot predict organisational events with complete or even probable certainty, what can predictive algorithms do?
To answer this, we must understand how they work. Once developed and inscribed with their base code, predictive algorithms need to be “trained” to hone their predictive power. This is done by feeding them with past organisational data. They then search for trends in the data and extrapolate rules that can be applied to future data.
For example, workforce planning algorithms can identify employees who are likely to resign. They do this by analysing the personality and behavioural patterns of employees who have resigned in the past and cross-referencing the results with the profiles of existing employees to identify those with the highest matching scores. With each round of application, the algorithm is continually adjusted to correct ever-decreasing prediction errors.
Read more: Why marking essays by algorithm risks rewarding the writing of 'bullshit'
However, the term “prediction error” is misleading because these algorithms do not predict, but rather extrapolate. All that predictive algorithms can ever do is guess at what is going to happen based on what has already happened. The leap required to make actual predictions is not a matter of computing power, but rather of bending the laws of physics.
Predictive models can’t anticipate change
Because they are extrapolative, predictive models are rather good at identifying regularities, continuity and routine. However, the human brain is also designed to identify stable patterns. Competent managers should be well aware of their organisation’s operations, and capable of envisioning steady patterns over time.
What managers find difficult to predict is change. Unfortunately, predictive models are also poor predictors of change. The more radical change is – different from existing patterns – the more poorly predicted it will be.
To manage effectively and develop their knowledge of current and likely organisational events, managers need to learn to build and trust their instinctual awareness of emerging processes rather than rely on algorithmic promises that cannot be realised. The key to effective decision-making is not algorithmic calculations but intuition.
Authors: Uri Gal, Associate Professor in Business Information Systems, University of Sydney