From robodebt to racism: what can go wrong when governments let algorithms make the decisions
- Written by Monika Sarder, Senior Strategic Analyst, Monash University
Algorithmic decision-making has enormous potential to do good. From identifying priority areas for first response after an earthquake hits, to identifying those at risk of COVID-19 within minutes, their application has proven hugely beneficial.
But things can go drastically wrong when decisions are trusted to algorithms without ensuring they adhere to established ethical norms. Two recent examples illustrate how government agencies are failing to automate fairness.
1. The algorithm doesn’t match reality
This problem arises when a one-size-fits-all rule is implemented in a complex environment.
The most recent devastating example was Australia’s Centrelink “robodebt” debacle. In that case, welfare payments made on the basis of self-reported fortnightly income were cross-referenced against an estimated fortnightly income, taken as a simple average of annual earnings reported to the Australian Tax Office, and used to auto-generate debt notices without any further human scrutiny or explanation.
This assumption is at odds with how Australia’s highly casualised workforce is actually paid. For example, a graphic designer who was unable to find work for nine months of the financial year but earned A$12,000 in the three months before June would have had an automated debt raised against her. This is despite no fraud having occurred, and this scenario constituting exactly the kind of hardship Centrelink is designed to address.
The scheme ultimately proved to be a disaster for the Australian government, which must now pay back an estimated A$721 million in wrongly issued debts after the High Court ruled the scheme unlawful. More than 470,000 debts were wrongfully raised by the scheme, primarily against low income earners, causing significant distress.
Read more: We need human oversight of machine decisions to stop robo-debt drama
2. Inputs embed racism
The stunning scenes of police violence in US cities have underscored the extent to which systemic racism influences law and order processes in the United States, from police patrols right through to sentencing. Black individuals are more likely to be stopped and searched, more likely to be arrested for low-level infractions, more likely to have prison time included in plea deals, and incur longer sentences for comparable crimes when they do go to trial.
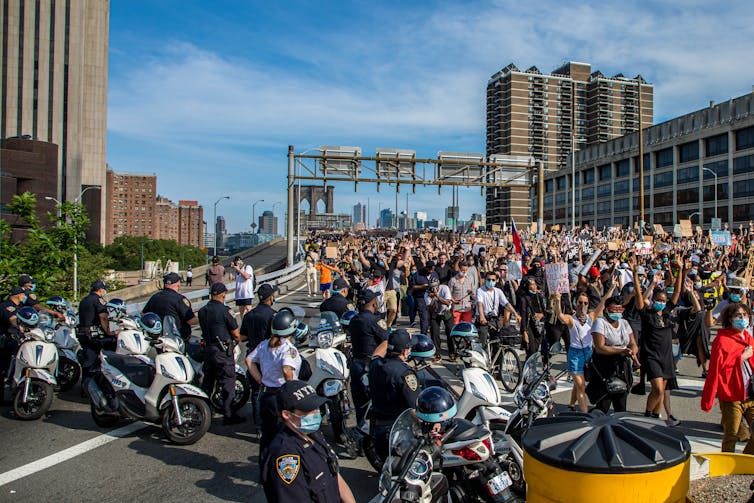
This systemic racism has been repeated, more insidiously, in algorithmic processes. One example is COMPAS, a controversial “decision support” system designed to help parole boards in the United States decide which prisoners to release early, by providing a probability score of their likelihood of reoffending.
Rather than rely on a simple decision rule, the algorithm used a range of inputs, including demographic and survey information, to derive a score. The algorithm did not use race as an explicit variable, but it did embed systemic racism by using variables that were shaped by police and judicial biases on the ground.
Applicants were asked a range of questions about their interactions with the justice system, such as the age they first came in contact with police, and whether family or friends had previously been incarcerated. This information was then used to derive their final “risk” score.
As Cathy O'Neill put it in her book Weapons of Math Destruction: “it’s easy to imagine how inmates from a privileged background would answer one way and those from tough inner streets another”.
What is going wrong?
Using algorithms to make decisions isn’t inherently bad. But it can turn bad if the automated systems used by governments fail to incorporate the principles real humans use to make fair decisions.
People who design and implement these solutions need to focus not just on statistics and software design, but also ethics. Here’s how:
consult those who are likely to be significantly affected by a new process before it is implemented, not after
check for potential unfair bias at the process design phase
ensure the underpinning rationale of the decisions is transparent, and the outcomes are relatively predictable
make a human accountable for the integrity of decisions and their consequences.
Read more: Algorithms are everywhere but what will it take for us to trust them?
It would be ideal if the developers of social policy algorithms put these principles at the core of their work. But in the absence of accountability in the tech sector, numerous laws have been passed, or are being passed, to deal with the problem.
The European Union data protection law states that algorithmic decisions that have significant consequences for any person must involve a human review component. It also requires organisations to provide a transparent explanation of the logic used in algorithmic processes.
The US Congress, meanwhile, is considering a draft Algorithmic Accountability Act that would require institutions to consider “the risks that the automated decision system may result in or contribute to inaccurate, unfair, biased, or discriminatory decisions impacting consumers”.
Legislation is a solution, but it is not the best one. We need to develop and embed ethics and norms around decision-making into organisational practice. For this we need to boost the public’s data literacy, so they have the language to demand accountability from the tech giants to which we are all increasingly beholden.
A transparent and open approach is vital if we are to make the most of the technologies on offer in our data-rich world, while retaining our rights as citizens.
Authors: Monika Sarder, Senior Strategic Analyst, Monash University