Combining the facial recognition decisions of humans and computers can prevent costly mistakes
- Written by David White, Scientia Fellow, UNSW
After a series of bank robberies that took place in the US in 2014, police arrested Steve Talley. He was beaten during the arrest and held in maximum security detention for almost two months. His estranged ex-wife identified him as the robber in CCTV footage and an FBI facial examiner later backed up her claims.
It turned out Talley was not the perpetrator. Unfortunately, his arrest left him with extensive injuries, and led to him losing his job and a period of homelessness. Talley has now become an example of what can go wrong with facial identification.
These critical decisions rest on the ability of humans and computers to decide whether two images are of the same person or different people. Talley’s case shows how errors can have profound consequences.
My research focuses on how to improve the accuracy of these decisions. This can make society safer by protecting against terrorism, organised crime and identity fraud. And make them fairer by ensuring that errors in these decisions do not lead to people being wrongly accused of crimes.
Read more: DNA facial prediction could make protecting your privacy more difficult
Identifying unfamiliar faces
So just how accurate are humans and computers at identifying faces?
Most people are extremely good at recognising faces of people they know well. However, in all of the critical decisions outlined above, the task is not to identify a familiar face, but rather to verify the identity of an unfamiliar face.
To understand just how challenging this task can be, try it for your self: are the images below of the same person or different people?
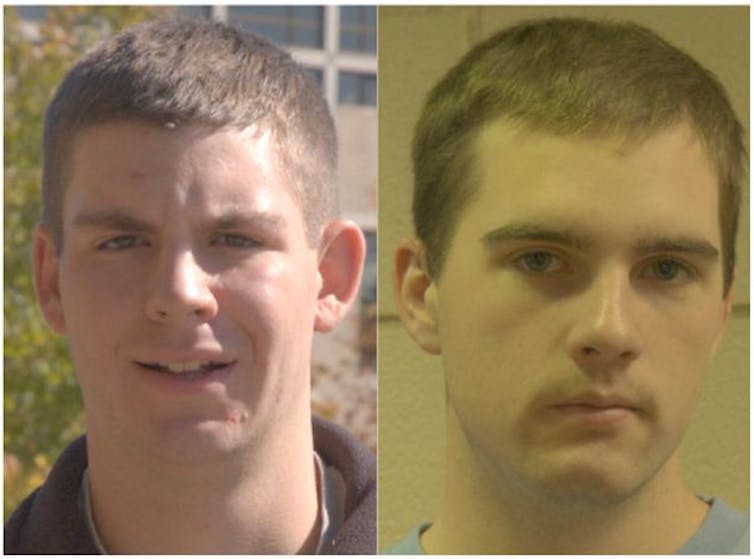
Humans versus machines
The above image pair is one of the test items my colleagues and I used to evaluate the accuracy of humans and computers in identifying faces, in a paper published last week in Proceedings of the National Academy of Science.
We recruited two groups of professional facial identification experts. One group were international experts that produce forensic analysis reports for court (Examiners). Another group were face identification specialists that made quicker decisions, for example when reviewing the validity of visa applications or in forensic investigation (Reviewers). We also recruited a group of “super-recognisers” who have a natural ability to identify faces, similar to groups that have been deployed as face identification specialists in the London Metropolitan Police.
Performance of these groups compared to undergraduate students and to the algorithms is shown in the graph below.
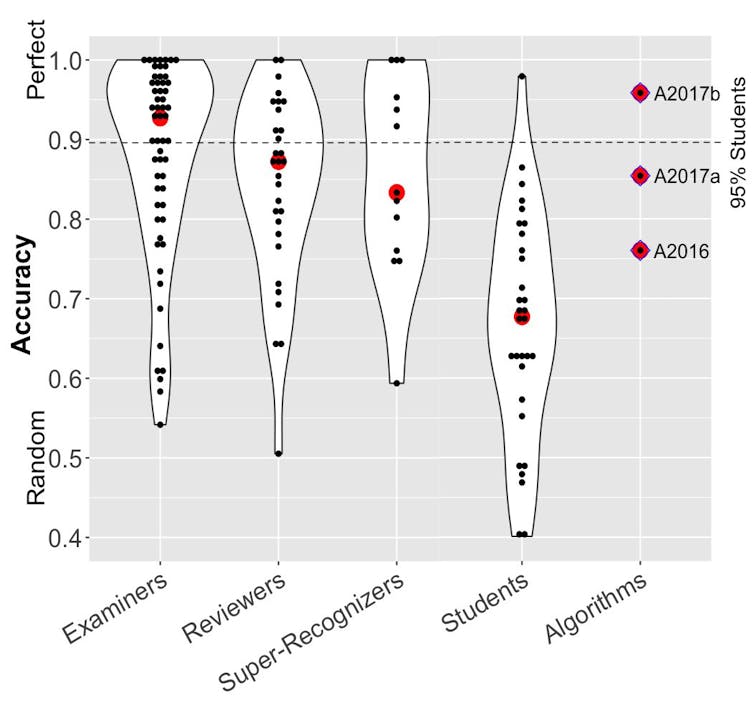
Black dots on this graph show the accuracy of individual participants, and the red dots show the average performance of the group.
The first thing to notice is that there is a clear ordering of performance across the groups of humans. Students perform relatively poorly as a group – with over 30% errors on average – showing just how challenging the task is.
The professional groups fare far better on the task, making less than 10% errors on average and nine out of 87 attaining the maximum possible score on the test.
Interestingly, the super-recognisers also performed extremely well, with three out of 12 attaining the maximum possible score. These people had no specialist training or experience in performing face identification decisions, suggesting that selecting people based on natural ability is also a promising solution.
Read more: Class action against Facebook over facial recognition could pave the way for further lawsuits
Performance of the algorithms is shown by the red dots on the right of the graph. We tested three iterations of the same algorithm as the algorithm was improved over the last two years. There is a clear improvement of this algorithm with each iteration, demonstrating the major advances that Deep Convolutional Neural Network technology have made over the past few years.
The most recent version of the algorithm attained accuracy that was in the range of the very best humans.
The wisdom of crowds
We also observed large variability in all groups. No matter which group we look at, performance of individuals spans the entire measurement scale – from random guessing (50%) to perfect accuracy (100%).
This variation is problematic, because it is individuals that provide face identification evidence in court. If performance varies so wildly from one individual to the next, how can we know that their decisions are accurate?
Our study provides a solution to this problem. By averaging the responses of groups of humans, using what is known as a “wisdom of crowds” approach, we were able to attain near-perfect levels of accuracy. Group performance was also more predictable than individual accuracy.
Perhaps the most interesting finding was when we combined the decisions of humans and machines.
By combining the responses of just one examiner and the leading algorithm, we were able to attain perfect accuracy on this test – better than either a single examiner or the best algorithm working alone.
Face recognition in Australia
This is a timely result as Australia rolls out the National Face Identification scheme, which will enable police agencies to search large databases of images using face recognition software.
Read more: Close up: the government's facial recognition plan could reveal more than just your identity
Importantly, this application of face recognition technology is not automatic – like automated border control systems are. Rather, the technology generates “candidate lists” like the one shown below. For the systems to be of any use, humans must review these candidate lists to decide if the target identity is present.
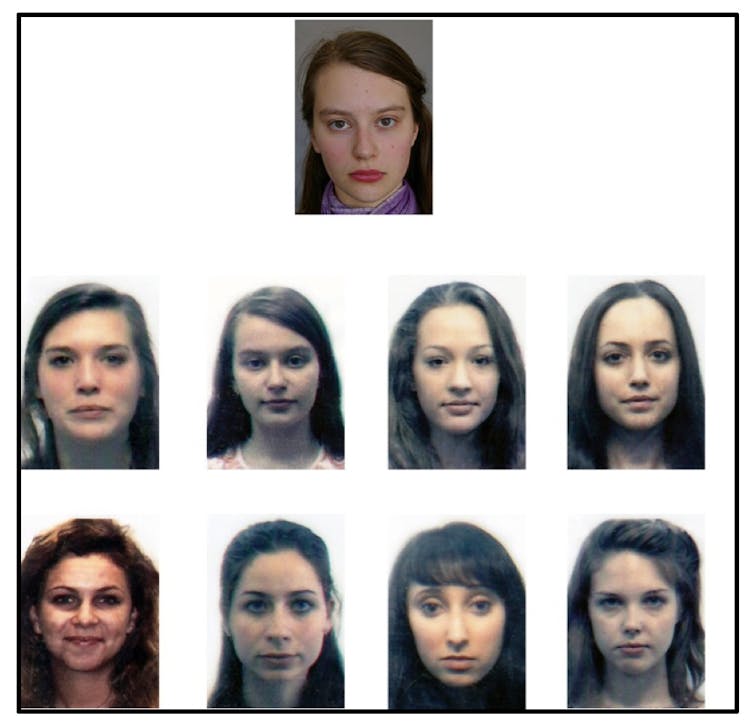
In a 2015 study my colleagues and I found that the average person makes errors on one in every two decisions when reviewing candidate lists, and chooses the wrong person 40% of the time!
False positives like these can waste precious police time, and have potentially devastating effect on people’s lives.
The study we published this week suggests that protecting against these costly errors requires careful consideration of both human and machine components of face recognition systems.
Correct answers: The pair of images are different people. The matching image in the candidate list is top row, second from left.
Authors: David White, Scientia Fellow, UNSW